Other areas in the Sokoban for the Mac web site: |
Sokoban home page |
Download More Levels |
Praise for Sokoban |
Read Documentation |
Sokoban for other Computers |
Papers about Sokoban |
Scott's home page |
Scott's other Mac programs |
Sokoban is a puzzle game requiring logical thinking andpuzzle-solving, not lightning-fast reflexes. The goal is to pushboxes to their correct destinations in a crowded warehouse. Thechallenge comes in having to think ahead; in the process of movingthe first box, you might accidently push another one into a corner.Because the boxes are so heavy, you can only push one at a time andcan't pull them at all.
1 Welcome to the Mac OS X Installation Guide This document describes the procedure for installing the Mac OS X FTDIUSBSerial driver for FTDI's FT2232C, FT232BM, FT245BM, FT8U232AM and FT8U245AM devices. The driver is available for free download from the Drivers section of the FTDI€web€site. This Macintosh version is completely free, and runs on any Mac under System 6.0.7 or later (finally including Mac OS X). Finally for OS X. Jan 26, 2006: Egad, it was 1.5 years ago that I threw this together. Anyway, feel free to try out the (preliminary) OS X version. Requires Mac OS X 10.2. Let me know how it works and inspire me to finish it up!
The game was invented in the early 1980s in Japan and won acomputer game contest there. Since then, versions have been writtenfor most personal computers.
This Macintosh version is completely free, andruns on any Mac under System 6.0.7 or later (finally including Mac OS X).
Finally for OS X
Jan 26, 2006: Egad, it was 1.5 years ago that I threw this together.Anyway, feel free to try out the (preliminary) OS X version. RequiresMac OS X 10.2. Let me know how it works and inspire me to finish it up!Download Sokoban for OS X.
Sokoban 2.5 beta!
Dec 20, 2001: A beta version of Sokoban 2.5 (for Mac OS 7, 8, 9, and Classic under OS X). This update allowslarger levels and lets you resize the main window.Download now(314K).
This beta may actually end up being the final version. I'm just callingit beta because I haven't tested it completely yet.
Sokoban for the Macintosh features
- Completely Free!
- Over 200 levels included
- Able to undo 10,000 moves
- Drag-and-drop movement lets the computer do the easy but boring stuff while you concentrate on the puzzle.
- Can make your own levels
- Fancy color or monochrome graphics
- Single-key undo (ESC key or various others instead of cmd-Z)
- Saves your solutions and lets you review them any time.
- Uses Macintosh Drag and Drop to make level editing much easier.
- You can quit in the middle of a level and pick up again where you left off.
Download
Download version 2.4.2a (202K; released April 11, 1999) athttp://sneezingtiger.com/sokoban/sokoban2.4.2a.hqx.
New features in version 2.4.2:
- Updated my address.
- Included Yoshio's levels (also available on Sokoban Levels page).
- Removed Boxxle and IQ Carrier levels because they are copyrighted and still being sold in Japan.
What was new in version 2.4:
- Better shortcuts: spacebar as a shortcut for Redo.
- Undo a whole action; for example, you can undo an entire drag move by hitting undo once instead of once for each step the pusher took in the course of that move.
- Sound support.
- Save solutions and remember solved levels in external collections (user-created text files).
Future Plans
At one time, I was working on a version 3.0, but the toolkit I was using isn't supported on OS X. Eventually, I'll learn enough about OS X programming to rewrite it there. But if you still have an old Mac and want totry a preliminary version, follow this link.
I also hope to find and distribute many new levels and encourage playersto create their own levels. Check theSokoban Levels page for new levels.
To be notified of updates to sokoban and these web pages,addyourself to my announcement list. You'll receive one email messageevery couple of months with notice about updates to Sokoban or these webpages, or opportunities for beta testing new versions.
Last modified: Jan 26, 2006.
Sokoban home page |Download More Levels |Praise for Sokoban |Read Documentation |Sokoban for other Computers |Papers about Sokoban |Scott's home page |Scott's other Mac programs
LOCOlib implements the LOCO and DUAL-LOCO algorithms for distributed statistical estimation.
Given a data matrix ( mathbf{X} in mathbb{R}^{ntimes p} ) and response ( mathbf{y} in mathbb{R}^n ), LOCO is a LOw-COmmunication distributed algorithm for ( ell_2 ) - penalised convex estimation problems of the form
[ min_{boldsymbol{beta}} J(boldsymbol{beta}) = frac{1}{n} sum_{i=1}^n f_i(boldsymbol{beta}^top mathbf{x}_i) + frac{lambda}{2} Vert boldsymbol{beta} Vert^2_2 ]
LOCO is suitable in a setting where the number of features ( p ) is very large so that splitting the design matrix across features rather than observations is reasonable. For instance, the number of observations ( n ) can be smaller than ( p ) or on the same order. LOCO is expected to yield good performance when the rank of the data matrix ( boldsymbol{X} ) is much lower than the actual dimensionality of the observations ( p ).
- Examples
- LOCOlib options
- Choosing the projection dimension
- Preprocessing package
- Data Structures
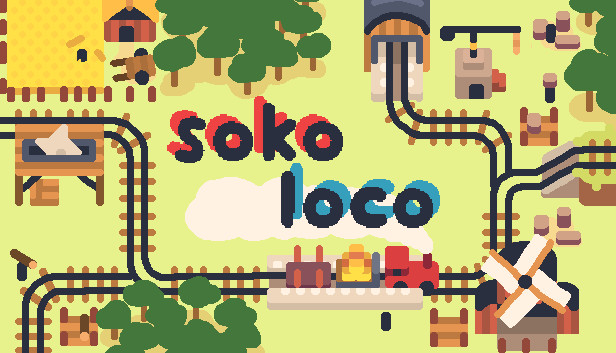
One large advantage of LOCO over iterative distributed algorithms such as distributed stochastic gradient descent (SGD) is that LOCO only needs one round of communication before the final results are sent back to the driver. Therefore, the communication cost – which is generally the bottleneck in distributed computing – is very low.
LOCO proceeds as follows. As a preprocessing step, the features need to be distributed across processing units by randomly partitioning the data into K blocks. This can be done with 'preprocessingUtils' package. After this first step, some number of feature vectors are stored on each worker. We shall call these features the 'raw' features of worker (k). Subsequently, each worker applies a dimensionality reduction on its raw features by using a random projection. The resulting features are called the 'random features' of worker (k). Then each worker sends its random features to the other workers. Each worker then adds or concatenates the random features from the other workers and appends these random features to its own raw features. Using this scheme, each worker has access to its raw features and, additionally, to a compressed version of the remaining workers' raw features. Using this design matrix, each worker estimates coefficients locally and returns the ones for its own raw features. As these were learned using the contribution from the random features, they approximate the optimal coefficients sufficiently well. The final estimate returned by LOCO is simply the concatenation of the raw feature coefficients returned from the ( K ) workers.
LOCO's distribution scheme is illustrated in the following figure.
A preprocessing step is required to distribute the data across workers according to the features rather than the observations. For this step, we provide the package 'preprocessingUtils'.
Building with sbt
Checkout the project repository
and build the packages with
and
To install sbt
on Mac OS X using Homebrew, run brew install sbt
. On Ubuntu run sudo apt-get install sbt
.
Running on Windows
To run LOCO locally under Windows, we recommend using Spark 1.3.1, download winutils.exe, move it to DISK:FOLDERSbin
and set HADOOP_CONF=DISK:FOLDERS
.
Ridge Regression
To run ridge regression locally on the ‘climate' regression data set provided here, unzip climate-serialized.zip
into the data
directory, download a pre-build binary package of Spark, set SPARK_HOME
to the location of the Spark folder, cd
into loco-lib/LOCO and run:
The estimated coefficients can be visualised as follows as each feature corresponds to one grid point on the globe. For more information on the data set, see LOCO: Distributing Ridge Regression with Random Projections.
SVM
To train a binary SVM with hinge loss locally on the ‘dogs vs. cats' classification data set provided here, first preprocess the text file with the preprocessingUtils package (see below) and run:
The following list provides a description of all options that can be provided to LOCO.
outdir
Directory where to save the summary statistics and the estimated coefficients as text files
saveToHDFS
True if output should be saved on HDFS
readFromHDFS
True if preprocessing was run with saveToHDFS
set to True
nPartitions
Number of blocks to partition the design matrix
nExecutors
Number of executors used. This information will be used to determine the tree depth in treeReduce
when the random projections are added. A binary tree structure is used to minimise the memory requirements.
trainingDatafile
Path to the training data files (as created by preprocessingUtils package)
testDatafile
Path to the test data files (as created by preprocessingUtils package)
responsePathTrain
Path to response corresponding to training data (as created by preprocessingUtils package)
responsePathTest
Path to response corresponding to test data (as created by preprocessingUtils package)
nFeats
Path to file containing the number of features (as created by preprocessingUtils package)
seed
Random seed
useSparseStructure
True if sparse data structures should be used
classification
True if the problem at hand is a classification task, otherwise ridge regression will be performed
numIterations
Number of iterations used in SDCA
checkDualityGap
If SDCA is chosen, true if duality gap should be computed after each iteration. Note that this is a very expensive operation as it requires a pass over the full local data sets (no communication required). Should only be used for tuning purposes.
stoppingDualityGap
If SDCA is chosen and checkDualityGap
is set to true, duality gap at which optimisation should stop
projection
Random projection to use: can be either 'sparse' or 'SDCT'

One large advantage of LOCO over iterative distributed algorithms such as distributed stochastic gradient descent (SGD) is that LOCO only needs one round of communication before the final results are sent back to the driver. Therefore, the communication cost – which is generally the bottleneck in distributed computing – is very low.
LOCO proceeds as follows. As a preprocessing step, the features need to be distributed across processing units by randomly partitioning the data into K blocks. This can be done with 'preprocessingUtils' package. After this first step, some number of feature vectors are stored on each worker. We shall call these features the 'raw' features of worker (k). Subsequently, each worker applies a dimensionality reduction on its raw features by using a random projection. The resulting features are called the 'random features' of worker (k). Then each worker sends its random features to the other workers. Each worker then adds or concatenates the random features from the other workers and appends these random features to its own raw features. Using this scheme, each worker has access to its raw features and, additionally, to a compressed version of the remaining workers' raw features. Using this design matrix, each worker estimates coefficients locally and returns the ones for its own raw features. As these were learned using the contribution from the random features, they approximate the optimal coefficients sufficiently well. The final estimate returned by LOCO is simply the concatenation of the raw feature coefficients returned from the ( K ) workers.
LOCO's distribution scheme is illustrated in the following figure.
A preprocessing step is required to distribute the data across workers according to the features rather than the observations. For this step, we provide the package 'preprocessingUtils'.
Building with sbt
Checkout the project repository
and build the packages with
and
To install sbt
on Mac OS X using Homebrew, run brew install sbt
. On Ubuntu run sudo apt-get install sbt
.
Running on Windows
To run LOCO locally under Windows, we recommend using Spark 1.3.1, download winutils.exe, move it to DISK:FOLDERSbin
and set HADOOP_CONF=DISK:FOLDERS
.
Ridge Regression
To run ridge regression locally on the ‘climate' regression data set provided here, unzip climate-serialized.zip
into the data
directory, download a pre-build binary package of Spark, set SPARK_HOME
to the location of the Spark folder, cd
into loco-lib/LOCO and run:
The estimated coefficients can be visualised as follows as each feature corresponds to one grid point on the globe. For more information on the data set, see LOCO: Distributing Ridge Regression with Random Projections.
SVM
To train a binary SVM with hinge loss locally on the ‘dogs vs. cats' classification data set provided here, first preprocess the text file with the preprocessingUtils package (see below) and run:
The following list provides a description of all options that can be provided to LOCO.
outdir
Directory where to save the summary statistics and the estimated coefficients as text files
saveToHDFS
True if output should be saved on HDFS
readFromHDFS
True if preprocessing was run with saveToHDFS
set to True
nPartitions
Number of blocks to partition the design matrix
nExecutors
Number of executors used. This information will be used to determine the tree depth in treeReduce
when the random projections are added. A binary tree structure is used to minimise the memory requirements.
trainingDatafile
Path to the training data files (as created by preprocessingUtils package)
testDatafile
Path to the test data files (as created by preprocessingUtils package)
responsePathTrain
Path to response corresponding to training data (as created by preprocessingUtils package)
responsePathTest
Path to response corresponding to test data (as created by preprocessingUtils package)
nFeats
Path to file containing the number of features (as created by preprocessingUtils package)
seed
Random seed
useSparseStructure
True if sparse data structures should be used
classification
True if the problem at hand is a classification task, otherwise ridge regression will be performed
numIterations
Number of iterations used in SDCA
checkDualityGap
If SDCA is chosen, true if duality gap should be computed after each iteration. Note that this is a very expensive operation as it requires a pass over the full local data sets (no communication required). Should only be used for tuning purposes.
stoppingDualityGap
If SDCA is chosen and checkDualityGap
is set to true, duality gap at which optimisation should stop
projection
Random projection to use: can be either 'sparse' or 'SDCT'
nFeatsProj
Projection dimension
concatenate
True is random projections should be concatenated, otherwise they are added. The latter is more memory efficient.
CV
If true, performs cross validation
Mac Os Mojave
kfold
Number of splits to use for cross validation
lambdaSeqFrom
Start of regularisation parameter sequence to use for cross validation
lambdaSeqTo
End of regularisation parameter sequence to use for cross validation Infinity challenge mac os.
lambdaSeqBy
Step size for regularisation parameter sequence to use for cross validation
lambda
If no cross validation should be performed (CV=false
), regularisation parameter to use
Choosing the projection dimension
The smallest possible projection dimension depends on the rank of the data matrix ( boldsymbol{X} ). If you expect your data to be low-rank so that LOCO is suitable, we recommend using a projection dimension of about 1%-10% of the number of features you are compressing. The latter depends on whether you choose to add or to concatenate the random features. This projection dimension should be used as a starting point. Of course you can test whether your data set allows for a larger degree of compression by tuning the projection dimension together with the regularisation parameter ( lambda ).
Concatenating the random features
As described in the original LOCO paper, the first option for collecting the random projections from the other workers is to concatenate them and append these random features to the raw features. More specifically, each worker has ( tau = p / K ) raw features which are compressed to ( tau_{subs} ) random features. These random features are then communicated and concatenating all random features from the remaining workers results in a dimensionality of the random features of ( (K-1) cdot tau_{subs} ). Finally, the full local design matrix, consisting of raw and random features, has dimension ( n times (tau + (K-1) cdot tau_{subs}) ).
Adding the random features
Alternatively one can add the random features. This is equivalent to projecting all raw features not belonging to worker ( k ) at once. If the data set is very low-rank, this scheme may allow for a smaller dimensionality of the random features than concatenation of the random features as we can now project from ( (p - p/K)) to ( tau_{subs} ) instead of from ( tau = p/K ) to ( tau_{subs} ).
The preprocessing package ‘preprocessingUtils' can be used to
- center and/or scale the features and/or the response to have zero mean and unit variance, using Spark MLlib's
StandardScaler
. This can only be done when using a dense data structure for the features (i.e.sparse
must be set tofalse
). - save data files in serialised format using the Kryo serialisation library. This code follows the example from @phatak-dev provided here.
convert text files of the formats 'libsvm', 'comma', and 'space' (see examples under options) to object files with RDDs containing
observations of type
LabeledPoint
(needed for the algorithms provided in Spark's machine learning library MLlib)Array[Double]
where the first entry is the response, followed by the features
feature vectors of type
FeatureVectorLP
(needed for LOCO, see details below)
Data Structures
The preprocessing package defines a case class LOCO relies on:
FeatureVectorLP
The case class FeatureVector
contains all observations of a particular variable as a vector in the field observations
(can be sparse or dense). The field index
serves as an identifier for the feature vector.
Example
To use the preprocessing package
- to center and scale the features
- to split one data set into separate training and test sets
- to save the data sets as object files using Kryo serialisation, distributed over (a) observations and (b) features
download the ‘dogs vs. cats' classification data set provided here, unzip dogs_vs_cats.zip
into the data
directory,change into the corresponding directory with cd loco-lib/preprocessingUtils
and run:
preprocessingUtils options
The following list provides a description of all options that can be provided to the package ‘preprocessingUtils'.
outdir
Directory where to save the converted data files
saveToHDFS
True if output should be saved on HDFS
nPartitions
Number of partitions to use
dataFormat
Can be either 'text' or 'object'
sparse
True if sparse data structures should be used
textDataFormat
If dataFormat
is 'text', it can have the following formats:
- 'libsvm' : LIBSVM format, e.g.:
- 'comma' : The response is separated by a comma from the features. The features are separated by spaces, e.g.:
- 'spaces' : Both the response and the features are separated by spaces, e.g.:
dataFile
Path to the input data file
separateTrainTestFiles
True if (input) training and test set are provided in different files
trainingDatafile
If training and test set are provided in different files, path to the training data file
testDatafile
If training and test set are provided in different files, path to the test data file
proportionTest
If training and test set are not provided separately, proportion of data set to use for testing
seed
Random seed
outputTrainFileName
File name for folder containing the training data
outputTestFileName
File name for folder containing the test data
outputClass
Specifies the type of the elements in the output RDDs : can be LabeledPoint
or DoubleArray
Jetpack joyrider mac os.
twoOutputClasses
True if same training/test pair should be saved in two different formats
secondOutputClass
If twoOutputClasses
is true, specifies the type of the elements in the corresponding output RDDs
centerFeatures
True if features should be centred to have zero mean
centerResponse
True if response should be centred to have zero mean
scaleFeatures
True if features should be scaled to have unit variance
Mac Os Versions
Pendulis [preview] mac os. scaleResponse
True if response should be scaled to have unit variance
Soko Loco Mac Os Download
Note that the benefit of some of these setting highly depends on the particular architecture you will be using, i.e. we cannot guarantee that they will yield optimal performance of LOCO.
- Use Kryo serialisation
- Increase the maximum allowable size of the Kryo serialization buffer
- Use Java's more recent 'garbage first' garbage collector which was designed for heaps larger than 4GB if there are no memory constraints
- Set the total size of serialised results of all partitions large enough to allow for the random projections to be send to the driver
The LOCO algorithm is described in the following papers:
Mac Os Download
- Heinze, C., McWilliams, B., Meinshausen, N., Krummenacher, G., Vanchinathan, H. P. (2015) LOCO: Distributing Ridge Regression with Random Projections
- Heinze, C., McWilliams, B., Meinshausen, N. DUAL-LOCO: Distributing Statistical Estimation Using Random Projections AISTATS 2016
Soko Loco Mac Os Catalina
Further references:
- Shalev-Shwartz, S. and Zhang, T. Stochastic dual coordinate ascent methods for regularized loss minimization. JMLR, 14:567–599, February 2013c.